728x90
728x90
오늘은 딥러닝의 기본인 Tensorflow에 대해서 알아보자!!
일단 하기에 앞서 간단한 준비가 필요하다. 차근차근 해보자.
참고
가상 환경 준비
conda create -n 가상환경이름
- conda create -n tf1 python=3.7 anaconda
- activate 가상환경이름
- conda deactivate
설치
- pip install tensorflow
- pip install tensorflow==1.15.0
- conda install tensorflow==1.15.0
특징
- 구글에서 만든 오픈소스 머신러닝 프레임워크
- 다양한 언어를 제공하며 파이썬이 가장 많이 사용됨
- 기본적으로 텐서를 활용한 그래프 수치 연산을 하는 도구
머신러닝 프레임워크 종류들
- Theano
- Pytorch
- Torch
- Caffe
- Keras
- ...
기본 용어
- 그래프 : 수학적인 의미에서 노드와 엣지로 구성된 기하 모형
- 노드(node) : 연산 및 데이터 정의
- 엣지(edge) : 노드를 연결하는 선(데이터의 흐름)
- 텐서(tensor) : 다차원 데이터 배열
- 세션(session) : 그래프를 실행시키기 위해서 필요한 객체
우선 텐서플로우를 다운한다.
pip install tensorflow==1.15.0 as tf
import 시키고 버전을 확인한다.
import tensorflow as tf
import sys
print(sys.version)
print(tf.__version__)
3.7.9 (default, Aug 31 2020, 17:10:11) [MSC v.1916 64 bit (AMD64)]
1.15.0
1. 텐서
0D텐서
import numpy as np
# 0D 텐서(스칼라)
x=np.array(10)
x
x.ndim
x.shape
x.dtype
dtype('int32')
1D텐서
# 1D 텐서(스칼라)
x=np.array([10])
x
x.ndim
x.shape
x.dtype
dtype('int32')
2D텐서
# 2D 텐서()
x=np.array([[1,2,3,4],[5,6,7,8],[9,10,11,12]])
x
x.ndim
x.shape
(3, 4)
3D텐서, 고차원 텐서
# 3D 텐서, 고차원 텐서
# 딥러닝에서는 0D ~ 4D까지 주로 사용
# 동영상을 다룰때에는 5D 텐서까지 사용
# 자주 사용하는 속성
# 1. 축의 갯수(rank) : ndim
# 2. 크기 : shape
# 3. 타입 : dtype
2. 기본 구성 요소
- placeholder()
- constant()
- tf.constant(value, dtype=None, shape=None, name='Const', verify_shape=False)
- Variable()
- tf.Variable(initial_value=None, trainable=None, collections=None, validate_shape=True,caching_device=None, name=None, variable_def=None, dtype=None,expected_shape=None, import_scope=None, constraint=None,use_resource=None,synchronization=tf.VariableSynchronization.AUTO,aggregation=tf.VariableAggregation.NONE, shape=None )
1) constant
- 절대 변하지 않는 상수를 의미
- 자체적으로 그래프를 가진다,
- 메서드
a=tf.constant(10)
print(a)
b=tf.constant(20)
print(b)
c=tf.add(a,b)
print(c)
Tensor("Const_4:0", shape=(), dtype=int32)
Tensor("Const_5:0", shape=(), dtype=int32)
Tensor("Add_1:0", shape=(), dtype=int32)
sess = tf.Session()
print(sess.run(a))
print(sess.run(b))
print(sess.run(c))
10
20
30
# 결과 : 5*4*3*2*1
120
# TensorBoard
tf.summary.FileWriter("log_dir", graph=sess.graph)
# tensorboard -- logdir=log_dir
<tensorflow.python.summary.writer.writer.FileWriter at 0x251a9f8ca08>
sess.close()
#(5+2)*3
a=tf.constant([5])
b=tf.constant([2])
c=tf.add(a,b)
d=tf.constant([3])
e=tf.multiply(c,d)
sess = tf.Session()
sess.run(e)
array([21])
tf.summary.FileWriter("log_dir",graph=sess.graph)
<tensorflow.python.summary.writer.writer.FileWriter at 0x251a9f9ba88>
2) Variable
- 객체로 정의되어 있다.
- weight(가중치)를 저장하는 변수
- 사용 전에 반드시 초기화(variables_initializer()호출)
In [25]:
v1 = tf.Variable(initial_value=[5])
v2 = tf.Variable([3])
v3 = tf.Variable([2])
v4 = v1 + v2 + v3
print(v4)
Tensor("add_4:0", shape=(1,), dtype=int32)
sess = tf.Session()
sess.run(tf.global_variables_initializer())
sess.run(v4)
array([10])
tf.summary.FileWriter("log_dir",graph=sess.graph)
<tensorflow.python.summary.writer.writer.FileWriter at 0x251aad44108>
3)placeholder
- 그래프를 만들지 않는다.
- 입력된 데이터를 텐서와 매핑하는 역할
v1 = 5
v2 = 3
v3 = 2
p1=tf.placeholder(dtype=tf.float32)
p2=tf.placeholder(dtype=tf.float32)
p3=tf.placeholder(dtype=tf.float32)
result = p1+p2+p3
v1 = 5
v2 = 3
v3 = 2
sess = tf.Session()
sess.run(result, feed_dict={p1:v1,p2:v2,p3:v3})
10.0
지금까지의 내용으로 간단한 구구단 프로그램을 만들어 보자.
# 구구단 프로그램
def gugu(dan):
left = tf.placeholder(tf.int32)
right = tf.placeholder(tf.int32)
calc = tf.multiply(left, right)
sess = tf.Session()
for i in range(1,10):
result = sess.run(calc, feed_dict={left:dan, right:i})
print("{}*{}={}".format(dan, i, result))
sess.close()
gugu(7)
7*1=7
7*2=14
7*3=21
7*4=28
7*5=35
7*6=42
7*7=49
7*8=56
7*9=63
오늘도 이렇게 Python의 텐서플로우에 대해서 알아보았다!!
다음은 또 무엇에 대해서 알려줄까!?? 기대하시라~~
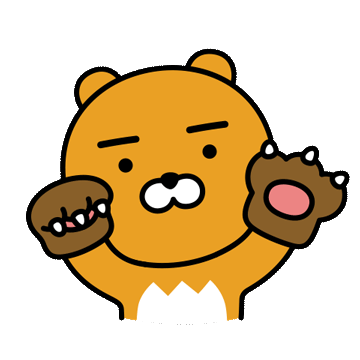
728x90
728x90
'Data Science' 카테고리의 다른 글
파이썬을 이용한 검색 알고리즘(선형, 이진) (0) | 2022.06.08 |
---|---|
파이썬을 이용한 알고리즘 (0) | 2022.06.07 |
R프로그래밍 Data Type 알아보기 (0) | 2022.06.03 |
파이썬을 이용한 AutoML 사용법 (0) | 2022.06.03 |
파이썬을 이용한 동적 크롤링 (0) | 2022.06.02 |
댓글